One of the biggest barriers to developing innovative digital capabilities in law enforcement is how to gain access to the right data for testing and training. More internet-connected devices, faster networks and more surveillance mean that we are all adding to our ballooning digital footprints every day. When it comes to investigations, law enforcement is faced with the overwhelming prospect of navigating these footprints in search of relevant information – in relation to victims as well as criminals. Therefore, it is essential that law enforcement knows how to sift through this data as efficiently and effectively as possible, particularly in time-critical situations.
Why is synthetic data needed?
Data protection laws, ethical concerns around data privacy and legislation preventing unnecessary intrusion mean that law enforcement cannot access large volumes of data, nor justify its use, for the purposes of testing and experimentation. Consequently, data scientists working in the field are very limited when it comes to understanding new types of data, or how new tools and techniques could be applied to give better insight. Not only are realistic volumes of data unavailable for test purposes, but using real data to explore new approaches introduce a whole host of ethical issues: What could such experimentation reveal about people whom the police are not officially investigating? What would happen if this data were leaked? Those challenges of collateral intrusion often present an insurmountable barrier to even trying.
To overcome this challenge, law enforcement is forced to turn to ‘fake’ or synthetic data for the purposes of experimentation and training, however realistic synthetic data is both very difficult and very time-consuming to create. Usually, the data will be created to mimic an investigative scenario but that would not be buried deep in the swathes of irrelevant data that investigators would need to assess and discard in real life, therefore removing a significant aspect of the challenge they face. Generally synthetic data is created manually and lacks the sophistication and complexity of a real-life scenario. For example, it may not account for the ways in which criminals will try to disguise their activity or how sudden absences in the data could be as meaningful as the data that actually is there.
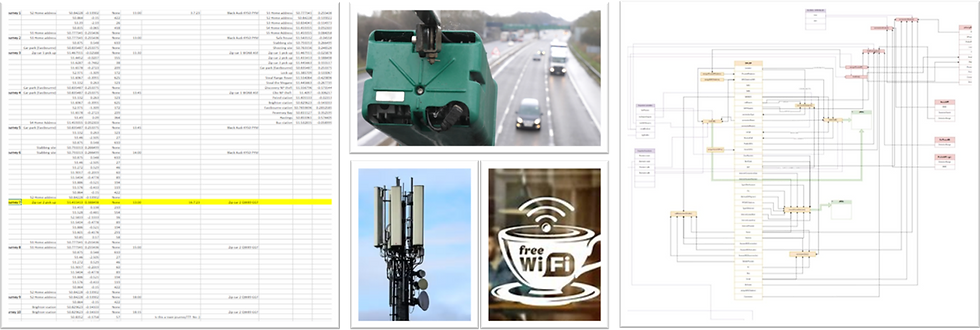
Our work with the Home Office’s Accelerated Capability Environment and other customers has shown the scale of the need to take a fresh approach to this problem, and who better to bring some fresh thinking than our summer interns? Drawn from a range of universities and academic disciplines, we set them the challenge of building a synthetic world, and the challenges they faced are described in more detail here. We’ve since refined this beyond the initial proof of concept into a synthetic data service, which can be tailored to meet different operational scenarios.
This generates data relevant to the investigation scenario and large volumes of background noise, mirroring what law enforcement is faced with in a real investigation. To use an age-old metaphor, it creates both the needle (the relevant data) and the haystack (the bulk data).
Step One: The ‘Needle’
The first step is the creation of an investigative scenario, that can be buried deep in our haystack of data. This could be wide-ranging and encompass a broad range of data types and we have experimented with missing persons, county lines networks and hostile reconnaissance ahead of a terrorist attack. Ensuring this scenario is as realistic as possible is key and relies heavily on the operational tradecraft that can only come from investigative experience. Once the scenario is understood, we can set some boundaries around generating our bulk data – for instance around the geographical setting, number of subjects of interest and the timeline for data generation.
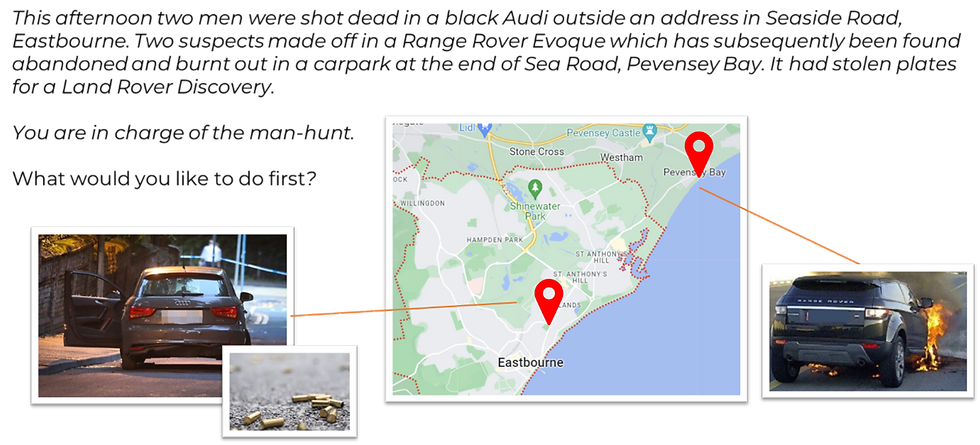
Step Two: The ‘Haystack’
The next step is to create the haystack, reflecting enough of the real world, against the parameters of the chosen operational scenario. This is effectively a dynamic world in which ‘agents’ made up of pedestrians, cyclists and drivers are set off on journeys and generate a range of data organically throughout their day - much like we do in real life. These journeys follow set patterns, for example commuting to and from work, schools runs and wider leisure journeys. Some vehicles are designated as taxis, and will make journeys throughout the day (or night). Some vehicles are lorries, and will make longer journeys from cities out towards coastal areas. Whilst simplified, the patterns are designed to be representative of how real people move through the world. This reduces the randomness of the data and creates realistic patterns of life.
The synthetic world which the agents travel through is essentially a map of the UK, covered in sensors such as ANPR (Automatic Number Plate Recognition), 5G masts, and Wi-Fi hotspots. The positioning of these sensors reflects reality as far as possible, for example the locations of 5G masts in the UK is open-source information which we have incorporated. Where this information is not openly available and may be sensitive (such as ANPR cameras), the networks in the synthetic world have been modelled on real networks and locations (including UK road networks, locations of coffee shop chains and so on). We have even introduced financial data, so agents can stop and purchase items from real locations.
Each car in the synthetic world has a driver, passengers and mobile phones associated with it, and as the car moves, it triggers sensors within a given radius. Such as the car ‘pinging’ ANPR cameras and phones connecting to 5G masts and WiFi hotspots as they progress along the route. Much like how in the real world our phones will connect to the nearest 5G mast (and switch masts) as we travel, or temporarily connect to a public WiFi hotspot when we pass a particular coffee shop chain, the phones in this synthetic world behave in the same way. Each time this happens, a record is created.
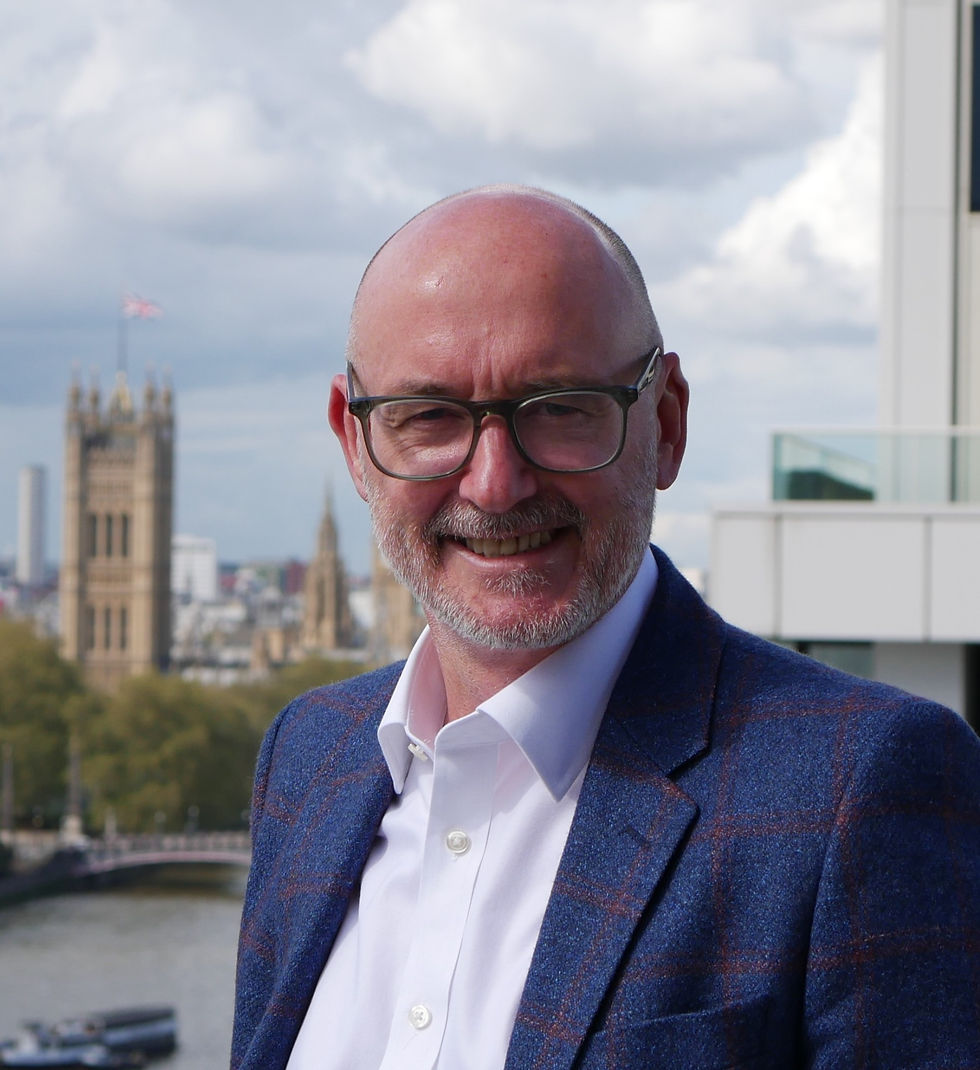
Phil Tomlinson, Principle One’s Digital Intelligence Lead explains our approach. “The scenario is handcrafted using a simple spreadsheet, which lets you input key entity information such as names, vehicles and phone numbers for your Subjects of Interest (SOIs) at specific dates and times. Essentially it lets you detail the movement of all SOIs in the case and upload the data ‘needles’ into the ‘haystack’. It can also be combined with non-criminal pattern of life data, to create a synthetic version of daily digital footprints. Finally, the data is uploaded into a series of files in the same formats used by real ANPR cameras, telecoms operators and Wi-Fi providers. This ensures the investigator or analyst is faced with the same challenges they would experience in a normal investigation, ie large volumes, data complexity, different formats and multiple sources.”
So let’s start the investigation!
The approach we have taken means that the outputs can be fed into any open-source or COTS data analysis tools and platforms without any concerns around privacy or intrusion. We’ve used tools provided by Elastic to rapidly analyse and visualise the data, rapidly focusing in on the subjects of interest around the relevant time period in question.
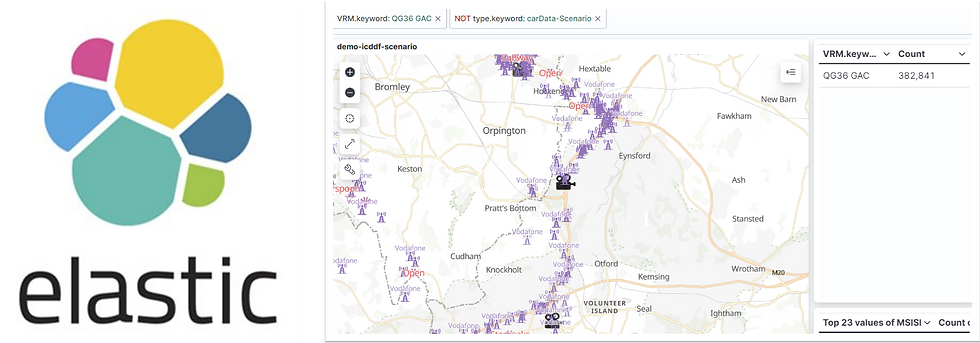
By isolating the entities that are associated with the subjects of interest very quickly through a geographical visualisation, it’s very easy to rapidly identify the sequence of events that took place and dig deeper into the data to identify connections between cars, mobile phones and other data to identify those who could be involved in the crime.
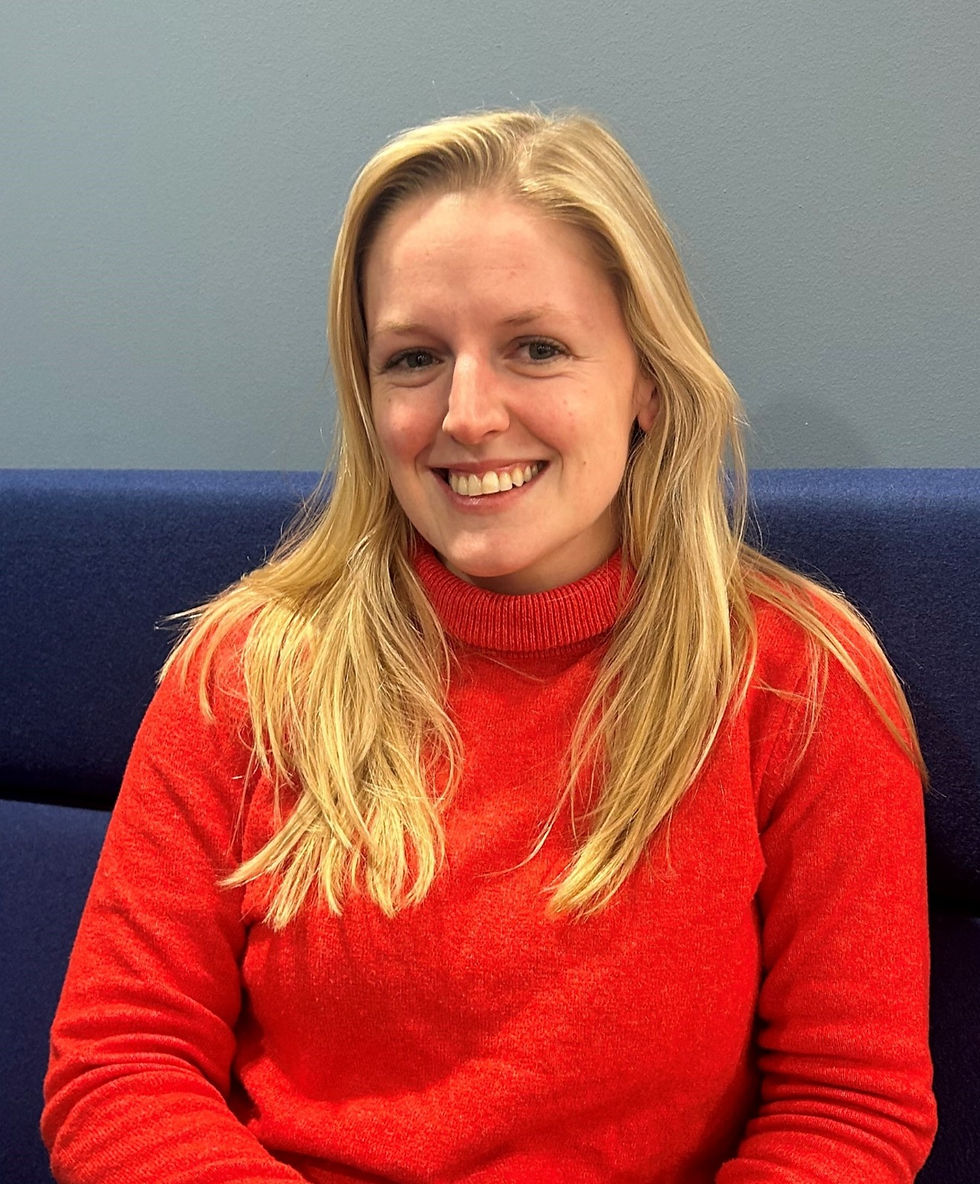
For Data Scientist, Dr Jess Flynn, this is a real accelerator for innovation. "My experience working within national security and law enforcement has shown time and time again that one of the biggest gaps when developing new capability is the lack of representative and dynamic test data. And with the buzz around Artificial Intelligence, Generative AI and Machine Learning, this is becoming an even more pressing need. The ability to create synthetic data that will test data exploitation products’ ability to find the ‘needle in the haystack’ within large volumes of data provides the opportunity to pro-actively build and tune tooling to meet the specific requirements and ultimately help data scientists access the right data for operational use at pace.”
As we continue to evolve the capability, we are also considering how we can add broader sources of data, to create a true multi-source capability. As the breadth of data available depends on each investigation, we are continuing to expand the library of scenarios that we are able to support ‘out of the box’ in addition to tailoring the capability against specific customer-provided investigations and can already see a wide range of benefits as a result:
Experiment and innovate with new tools and techniques to investigate crime without needing to operate within the constraints of legislation and data protection. Access to rich synthetic data will fast-track experimentation and innovation with 3rd party products;
Accelerate understanding of emerging data types: newer types of data may not be available at scale yet – creating them synthetically would allow law enforcement to ‘get on the front foot’ and explore them without needing to wait for larger quantities to trickle through;
Provide more realistic training: higher quality synthetic data would allow officers to learn more effectively in a low-risk environment, rather than being forced to learn ‘on the job’ as and when the right data presents itself. There are also opportunities to ‘gamify’ synthetic data and create realistic, immersive investigation scenarios which users could compete at (either with each other or themselves);
Influence legislation: experimentation with synthetic datasets in an ethical manner, which may not yet be available in the real world could enable law enforcement to identify new techniques for effective exploitation and thus influence changes to policy and legislation.
Through the data service we can create tailored, investigation-relevant synthetic data at a scale and pace that has not previously been possible. The value lies in how realistic it is, both in terms of volume and format, and the infinite possibilities that this creates for law enforcement to truly innovate, experiment and learn without constraints.
תגובות